How to Marketing Mix Modeling (MMM) with EXCEL — Intro
This article will help you understand what Marketing Mix Modeling is and how it can help your Business Grow. Thanks to Mike Taylor for his
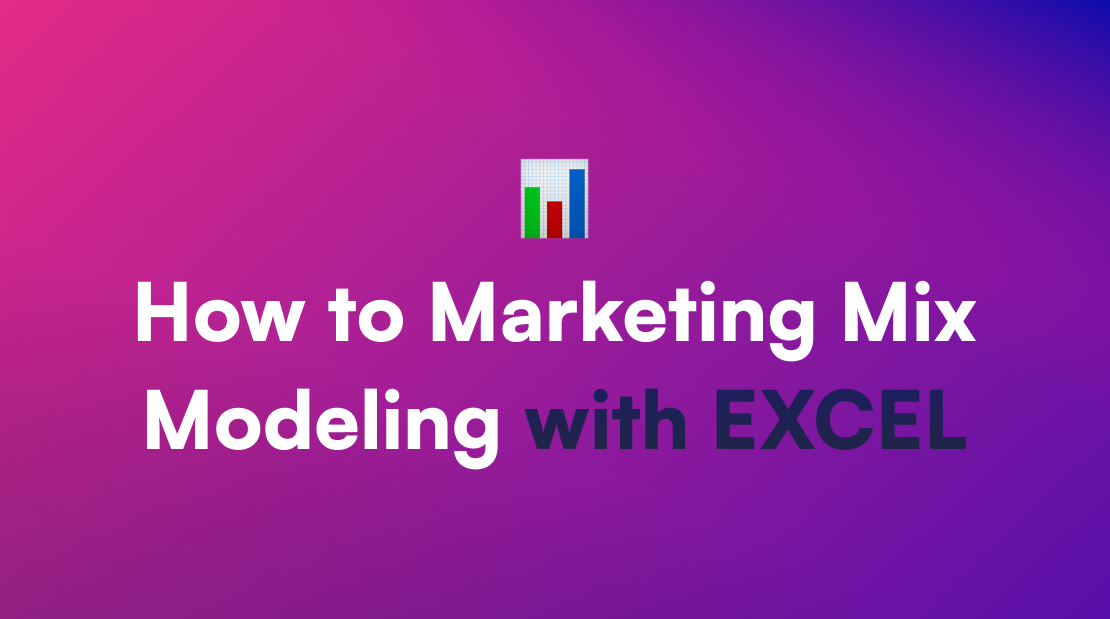
This article will help you understand what Marketing Mix Modeling is and how it can help your Business Grow.
Thanks to Mike Taylor for his post on Econometrics in GSheets, the inspiration for our template
If you prefer watching instead of reading, I’ve created a free Masterclass on Youtube: >>Click Here to Access<<
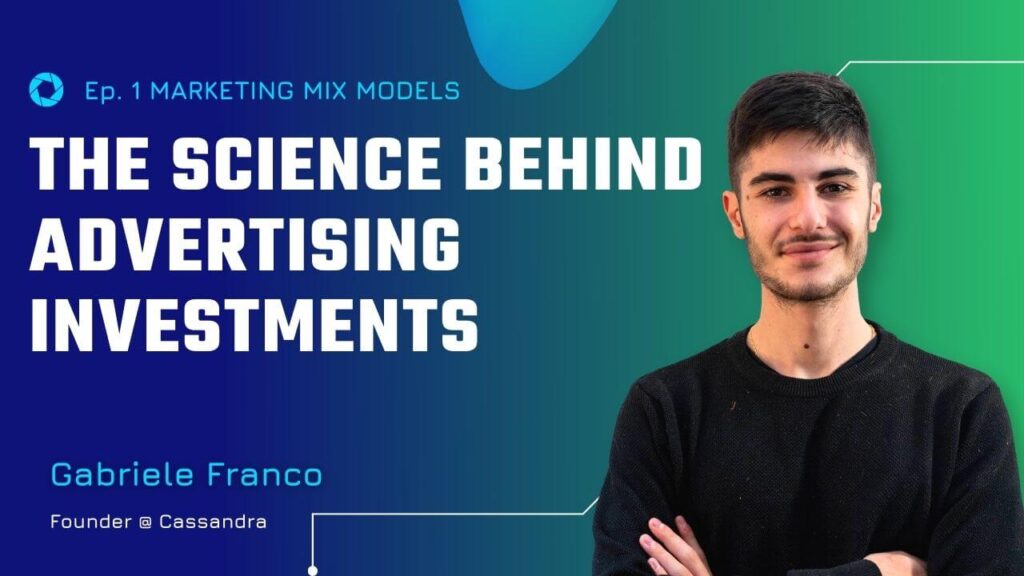
What is Marketing Mix Modeling
MMM are statistical techniques applied to historical data to understand the marginal contribution that each individual factor has on our sales.
They quantify the impact that each factor, measure what’s their contribution to sales and help you to understand how much to invest on each channel to maximize Sales or Conversions.
It’s an analytical approach invented in the 1960s and used by big corporations to allocate budgets across departments in order to achieve corporate financial goals.
In 2010, with the event of social media and search engines, MMM methodology has seen a downtrend… until now 😀
MTA vs MMM
In the eCommerce space, we are used to seeing Google analytics dashboards, that help us understand users’ behavior.
The analytical technology behind Google analytics is Multitouch Attribution (MTA).
It’s an analytical framework that uses cookies to track any user’s behavior inside our website.
It’s been incredibly powerful until April 2021 when IOS 14 have launched.
With IOS 14 there have been restrictions on sharing third-party cookies and this caused analytical tools that used cookies technology to have a high discrepancy with reality.
Facebook ads (Meta ads), Google ads, Google analytics, and many more platforms, have a really hard time tracking users’ behaviors.
While Multitouch Attribution focuses on the user’s behaviour, Marketing Mix Modeling focuses on how efficient is your investment
In fact, it’s a cookieless framework to do marketing analysis on your Marketing Mix, that hasn’t had any effect from IOS 14.
What is Marketing Mix Modeling Used for?
Marketing Mix Modeling (MMM) is used to approximate how many sales you are gonna have based on the values of your inputs:
Inputs can be:
- How much you spend on each channel
- What’s your product’s price
- Seasonality
- SEO Activities
- Competitor’s activities
- Offline media investments
- Other External factors
And from them we can:
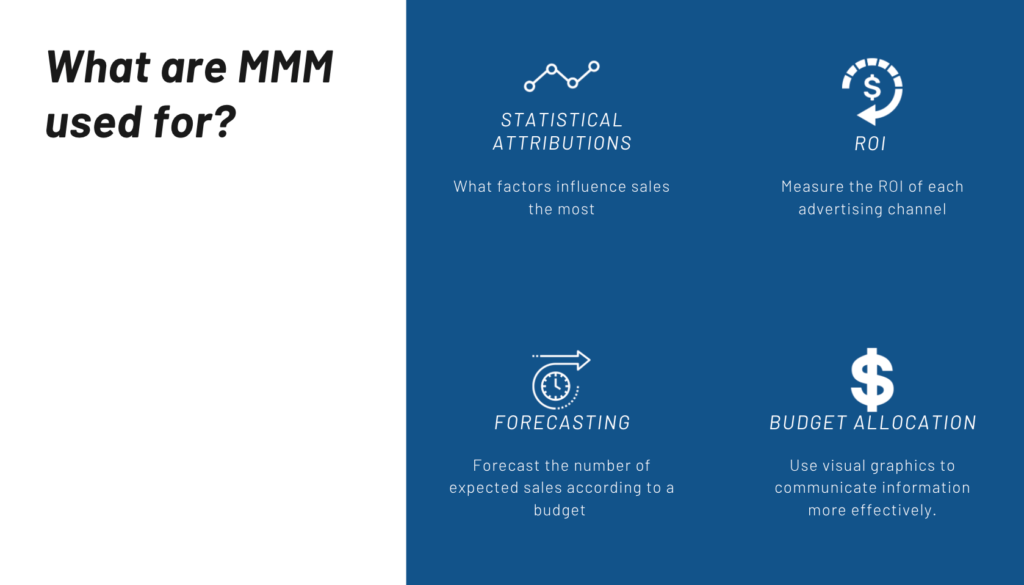
How do Marketing Mix Modeling (MMM) work in practice?
Let’s imagine we have a time series that described our sales over time
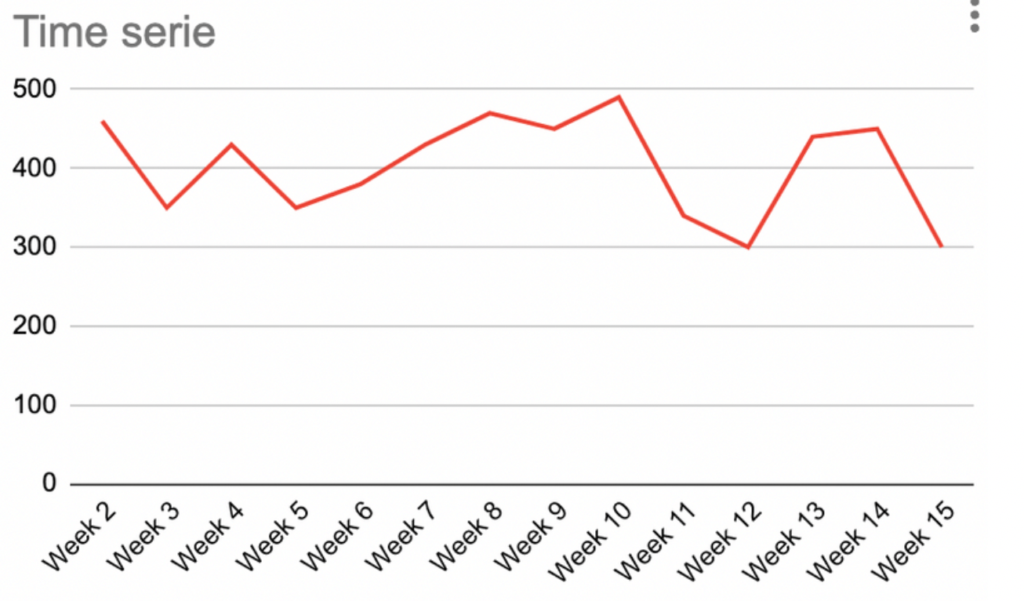
from this graph, we don’t understand enough, but what I notice are 2 spikes really close:
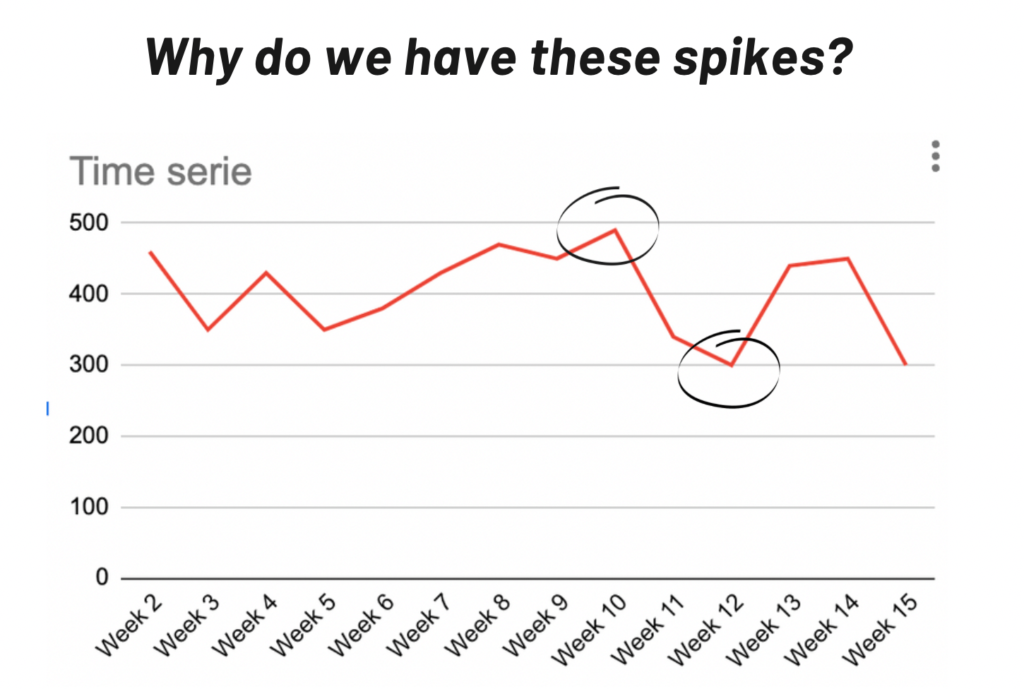
We are gonna use a Marketing Mix Modeling tool called MMM Decomposition graph to deduct what caused this variation.
In this graph, we have a decomposition of how Holiday, Ads Spent, TV spent, and price contributed to our weekly sales.

As we can clearly see the variation is described by the holiday’s contribution.
In the absence of “holiday”, we do 100$ less in sales.
The MMM decomposition graph it’s an incredible tool for Diagnostic Analysis and can help us understand why some variations happen.
What kind of data should I use in my Marketing Mix Modeling (MMM)?
Let’s explain the data engineering behind MMM dataset.
This is an example of a simple dataset for MMM:
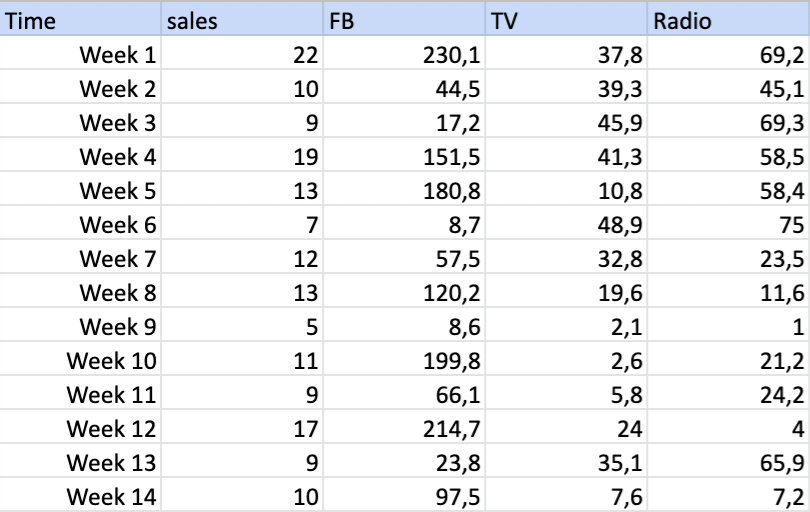
- First column: timestamp variable
- Second column: output variable (what you are trying to predict)
Can be either revenue, conversions, or even sessions.
- Other columns: input variables
There exist 2 types of input variables:
- Numerical: all the values that have an extended length from 0 to N.
They can be budget spent, sessions, price, impressions …
- Categorical: Input that has fixed values.
They can be a day of the week, months, year, promotion days …
They are generally described by boolean values (1 or 0)
If you are an eCommerce and you want to collect your data, follow this procedure:
- CMS: order export
Create a pivot on the orders with this framework:
Dimension: Date
Value: SUM(revenue) or SUM(orders)
- Adv Platform (report section):
Dimension: day or date (depending on the platform)
Value: Budget spent
- Google Analytics (report section):
Dimension: date
Value: Session
Filter: Medium: Organic
- Email Marketing (report section):
Dimension: date
Value: Recipient
Then copy and paste into multiple sheets in Google Sheets.
Use the “=VLOOKUP” function to centralize every value into the right cell in a unified sheet.
Repeat this process with all the sheets and data sources you have until you have one unified sheet with all your data.
MMM Transformation
Once we centralized everything we need to transform all advertising data with 2 transformations:
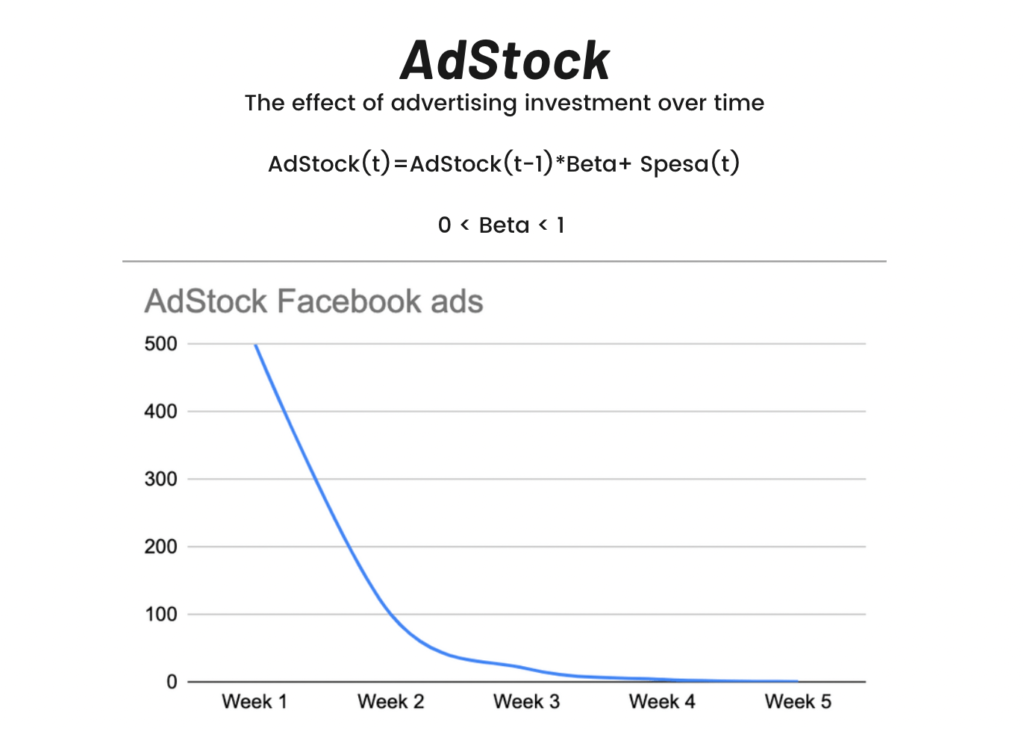
- AdStock: transformation that measures the impact of past advertisements on present sales.
It’s also called “Carryover effect”, and states that if you spend 10.000 € today, not all the people that see the ad will buy the same day, someone will buy the next day and someone else will buy the next week.
With the Adstock transformation, we approximate the marketing investment effect over time.

- Diminishing Returns: returns on investments are not linear, the more you increase your budget the less will be the marginal returns.
For this reason, we use a power function to approximate the response on advertising investments based on the volume invested.
>> See this EXCEL TEMPLATE to check all the formulas<<
Learning Algorithms
Once we transformed all the variables we can start using the function =Linest(‘output column’, ‘input columns’, 1, 1) (linear regression).
to get our model function.
Keep in mind that the linear regression creates a function similar to this one:
y = b1x1 + b2x2 + b3x3 + b0
It’s gonna give us the coefficients “b” of the function.
This is a Linear Regression’s output example on Excel
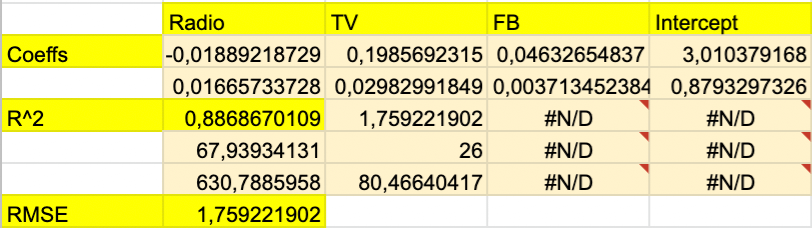
What this model is saying to us is:
Intercept = 3, which means if we shut down every media investment, we would still get 3 sales.
TV Coefficient = 0.19, means that every dollar spent on TV results in 0.19 incremental transactions
To generate 1 transaction with TV Spent, we would have to spend 5$.
Same School of thought for the other coefficients.
Hyperparametrization
After Training, the goal is to find the best performer hyperparameter for AdStock and diminishing returns that will increase our R²
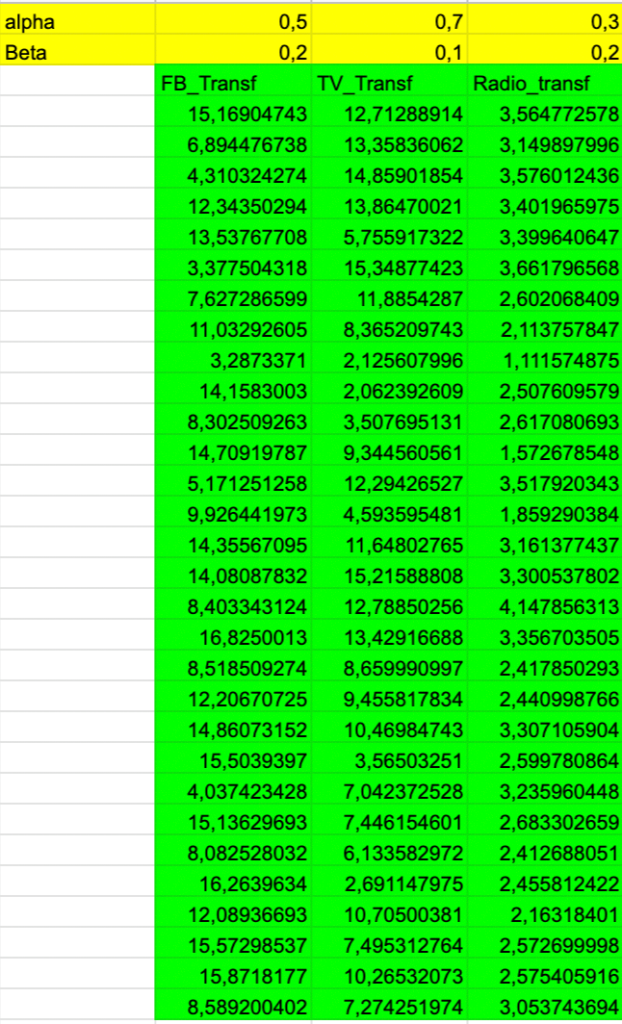
The values colored in yellow correspond to Beta and Alpha in the transformations we did before.
Transforming each media variable according to this formula:
media_transformed = (Adstock (t-1)*Beta + Spent(t))^Alpha
You can change them until you find all the values that maximize R².
I created a template that you can copy, so you’ll have the logic under your hand >> Click here to access it<<
Post-training analysis
After modeling, we need to generate our predictions and see graphically how accurate is our model.
The formula in excel would be something like this:
=Coef_FB$*B2+Coef_TV$*C2+Coef_Radio$*D2+Intercept$
You’ll find the actual formula in the excel template.
Results are gonna show our model predictions.
Copy the “actual sales” column next to predictions and create a line plot that shows how accurate are your predictions over time:
X-Axis = Time
Y-Axis = Prediction, Actual Sales
This would be the result
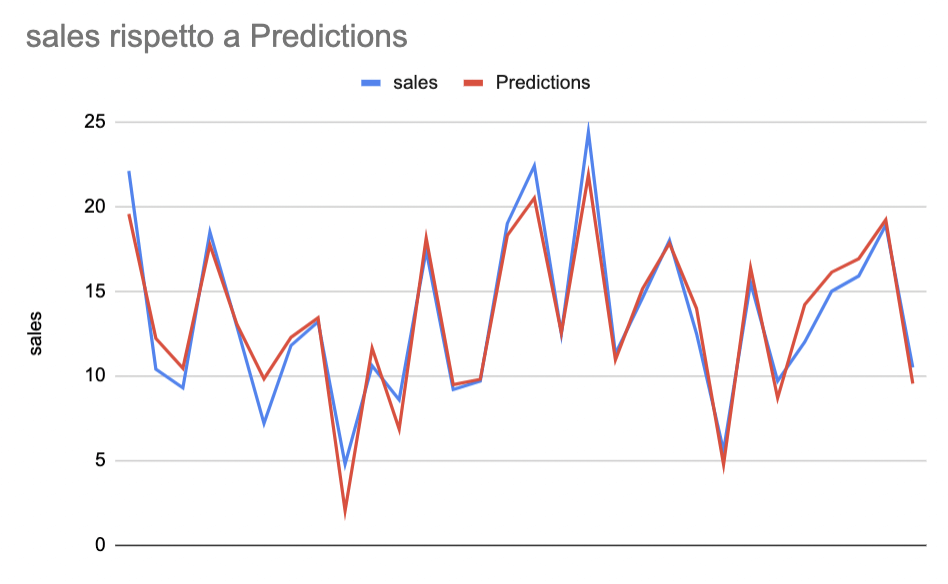
Conclusions
You created your first model on EXCEL, congratulations!
This would be just the introduction to Marketing Mix Modeling, in the next post we will cover:
- How to create a Forecaster Scenario Simulator
- How to create an automatic budget allocator
- How to do Diagnostic Analysis with a MMM Decomposition Graph
- How to see Statistical attributions from your model
Do you want to start now with Marketing Mix Modeling?
Discover Cassandra MMM builder
Cassandra is the first self-service software to model your media mix effectiveness on the cloud and receive media plans that maximize your sales.
Source: https://www.saxifrage.xyz/post/econometrics-gsheets