Analyzing the Latest Test Group Results: Key Findings and Insights
Discover key findings and insights from the latest test group results, including quantitative and qualitative analysis.
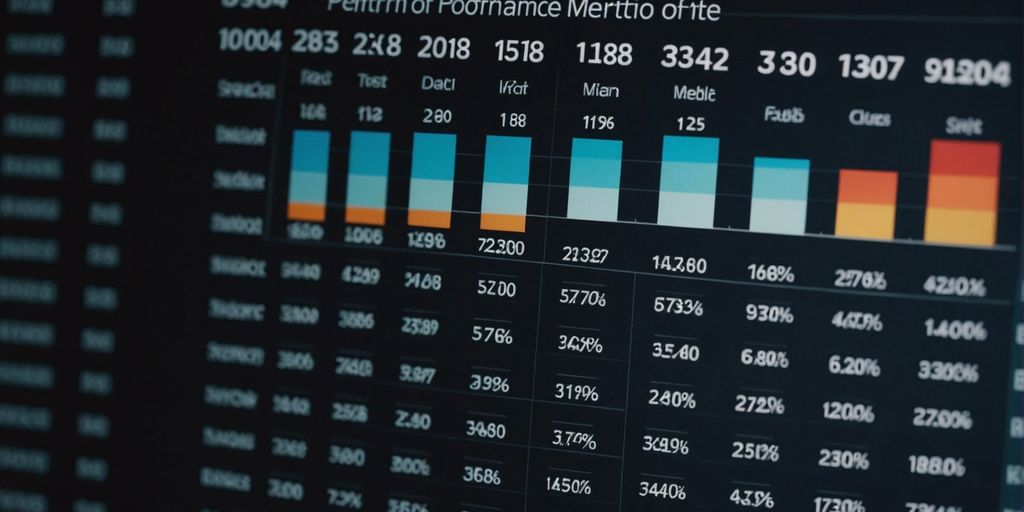
Analyzing the latest test group results is crucial for understanding user preferences, improving products, and guiding future experiments. By delving into both quantitative data and qualitative feedback, we can derive actionable insights that can drive meaningful changes. This article explores the importance of test group results, quantitative and qualitative analysis, common pitfalls, and how to apply these results to future experiments.
Key Takeaways
- Understanding both quantitative data and qualitative feedback is essential for a comprehensive analysis of test group results.
- Test group results help in formulating new hypotheses and guiding future experiments.
- Avoiding common pitfalls like confirmation bias and misinterpreting data is crucial for accurate analysis.
- Effective use of data visualization tools and statistical software can enhance the analysis process.
- Learning from both successful and failed tests can lead to continuous improvement and better decision-making.
Understanding the Importance of Test Group Results
Defining Test Group Results
Test Group Results are the outcomes derived from a controlled experiment where a subset of users is exposed to a variant while another subset (the control group) is not. These results are crucial for understanding user behavior and preferences. Accurately defining these results helps in making informed decisions that can lead to Conversion Rate Improvement and better user experience.
Why Test Group Results Matter
The significance of Test Group Results lies in their ability to provide actionable insights. By analyzing these results, businesses can identify what works and what doesn't, leading to Marketing ROI Optimization. For instance, if a new feature leads to a higher conversion rate in the test group compared to the control group, it indicates a positive impact. This can be quantified using metrics like Conversion Rate Optimization and Revenue Attribution.
Impact on Future Testing
Moreover, results from past tests can help your team come up with new hypotheses quickly. The team can identify the areas where the win from a past A/B test can be duplicated. Also, the team can look at failed tests, know the reason for their failure and steer clear of repeating mistakes. This iterative process is essential for Cross-Channel Measurement and Incrementality Testing, ensuring that each new test builds on the learnings from previous ones.
Analyzing your A/B test results is imperative, whether the outcome is positive, negative, or inconclusive. Delving deeper into these results provides validations specific to your users and helps in the overall digital marketing metrics strategy.
Quantitative Analysis of Test Group Results
Interpreting Numerical Data
Extracting hard numbers from the data is essential for effective quantitative data analysis. Figures like rankings and statistics will help you determine where the most common issues are on your website and their severity. For instance, metrics such as success rate and error rate can provide valuable insights. The success rate is the percentage of users in the testing group who ultimately completed the assigned task, while the error rate is the percentage of users that made or encountered the same error.
“The p-value for the comparison between the before and after groups of patients was .03% (Fig. 2), indicating that the greater the dissatisfaction among patients, the more frequent the improvements that were made to postoperative care.”
Identifying Winning Versions
After running the test, it's crucial to analyze the results to identify the winning version. This involves looking at both the quantitative data, like what version won the test, and the qualitative data, such as user feedback. For example, if you conducted an incrementality test on meta ads, you might find that one version revealed inflated attribution and higher cost per conversion.
Statistical Significance in Test Results
Understanding statistical significance is key to interpreting your test results accurately. A common method to present this kind of data is through a paired T-test table. For instance, if you find that 15 out of 60 patients in Group A responded negatively to a specific question, this data can help you make informed decisions. Statistical significance helps you determine whether the observed effects are due to chance or if they are genuinely impactful.
“As Figure 1 shows, 15 out of 60 patients in Group A responded negatively to Question 2.”
By focusing on these aspects, you can ensure that your quantitative analysis is both thorough and actionable.
Qualitative Insights from Test Group Feedback
Analyzing User Comments
Filtering through the feedback and comments is a good way to get an overall idea of how users felt about the product. If there are mostly positive comments, it means that the product is good. If there are mostly negative comments, it means that the product is not good. Observing the users while they are completing the tasks and taking notes on what they do and say can provide valuable insights. After the testing is complete, analyze the results by looking for patterns and common themes in the user feedback.
Identifying Positive Feedback
Include positive findings. In addition to the problems you've identified, include any meaningful positive feedback you received. This helps the team know what is working well so they can maintain those features in future website iterations. For example, if users consistently praise the ease of navigation, this is a feature worth keeping and enhancing.
Addressing Negative Feedback
Finding errors users had in the test is crucial. Look for patterns in the negative feedback to identify common issues. Once these issues are identified, they can be addressed in future iterations of the product. For instance, if multiple users report difficulty in finding a specific feature, this indicates a need for better usability design.
Qualitative data is just as, if not more, important than quantitative analysis because it helps to illustrate why certain problems are happening, and how they can be fixed. Such anecdotes and insights will help you come up with solutions to increase usability.
Common Pitfalls in Analyzing Test Group Results
Avoiding Confirmation Bias
Confirmation bias can significantly skew your analysis. It's crucial to approach data with an open mind and not just look for results that confirm your pre-existing beliefs. For example, if you believe a new feature will increase user engagement, you might overlook data that suggests otherwise. Instead, use Holdout Groups to compare and validate your findings objectively.
Misinterpreting Data
Misinterpreting data is a common issue that can lead to incorrect conclusions. Ensure you understand the context and the metrics you are analyzing. For instance, a spike in user activity might not necessarily mean increased engagement; it could be due to a temporary promotion. Always consider external factors and use tools like Attribution vs. Incrementality to get a clearer picture.
Overlooking Qualitative Feedback
Quantitative data is essential, but overlooking qualitative feedback can be a big mistake. User comments and feedback provide valuable insights that numbers alone can't offer. Conducting post-test segmentation can help you understand different user segments better. For example, analyzing user comments can reveal pain points that weren't evident from the data alone.
"No matter how the overall result of your A/B test turned out to be — positive, negative, or inconclusive — it is imperative to delve deeper and gather insights."
By keeping these pitfalls in mind, you can ensure a more accurate and comprehensive analysis of your test group results.
Applying Test Group Results to Future Experiments
Formulating New Hypotheses
Moreover, results from past tests can help your team come up with new hypotheses quickly. The team can identify the areas where the win from a past A/B test can be duplicated. Also, the team can look at failed tests, know the reason for their failure and steer clear of repeating mistakes.
Implementing Changes Based on Results
After you have analyzed the tests and documented them according to a predefined theme, make sure that you visit the knowledge repository before conducting any new test. For instance, you are developing a hypothesis for your product page, and want to test the product image size. Using a structured repository, you can easily find similar past tests which could help you understand patterns on that location.
Learning from Failed Tests
If this is your first year analyzing data, make these results the benchmark for your next analysis. Compare future results to this record and track changes over quarters, months, years, or whatever interval you prefer. You can even track data for specific subgroups to see if their experiences improve with your initiatives.
Bold: Make sure to document all findings meticulously to avoid repeating mistakes.
Conducting Post-Test Segmentation
You should also perform segmentation of your A/B tests and analyze them separately to get a clearer picture of what is happening. The results you derive from generic nonsegmented testing will provide illusory results that lead to skewed actions. There are broad types of segmentation that you can create to divide your audience. Here is a set of segmentation approach from Chadwick Martin Bailey:
- Demographic Segmentation
- Behavioral Segmentation
- Psychographic Segmentation
- Geographic Segmentation
Tools and Techniques for Effective Test Group Analysis
Using Data Visualization Tools
Data visualization tools are essential for interpreting complex test group results. They help transform raw data into understandable insights. Tools like Tableau and Power BI allow you to create interactive dashboards that can highlight key metrics and trends. For example, you can use a bar chart to compare the performance of different test groups or a line graph to track changes over time. These visualizations make it easier to identify patterns and outliers, facilitating more informed decision-making.
Leveraging Statistical Software
Statistical software such as SPSS, R, and Python libraries like Pandas and SciPy are invaluable for conducting in-depth analyses. These tools enable you to perform advanced statistical tests to determine the statistical significance of your results. For instance, you can use a t-test to compare the means of two groups or ANOVA for multiple groups. This helps in validating whether the observed differences are due to chance or a specific variable.
Best Practices for Data Collection
Effective data collection is the cornerstone of reliable test group analysis. Start by defining clear objectives and selecting appropriate metrics. Use tools like Google Analytics and Mixpanel to gather quantitative data, and consider conducting surveys or interviews for qualitative insights. Ensure your data is clean and well-organized to avoid skewed results. Segmenting your data can also provide a clearer picture of different user behaviors and preferences.
Conducting post-test segmentation can reveal insights that generic, non-segmented testing might miss, leading to more accurate actions.
Channel Impact Analysis
Understanding the impact of different marketing channels is crucial for optimizing your strategy. Use tools like Google Analytics to track the performance of various channels such as social media, email, and paid ads. By analyzing this data, you can identify which channels are driving the most conversions and allocate your budget more effectively. This is where Marketing Attribution Models come into play, helping you understand the contribution of each channel to your overall goals.
Marketing Attribution Models
Marketing Attribution Models are frameworks that help you assign credit to different marketing touchpoints. Common models include first-touch, last-touch, and multi-touch attribution. These models provide insights into the customer journey and help you understand which touchpoints are most effective. For example, a multi-touch attribution model can show you how different channels work together to drive conversions, offering a more holistic view of your marketing efforts.
Campaign Effectiveness
Measuring the effectiveness of your campaigns is essential for continuous improvement. Use KPIs such as click-through rates, conversion rates, and ROI to evaluate performance. Tools like Google Analytics and HubSpot can provide detailed reports on these metrics. By regularly reviewing these reports, you can identify areas for improvement and optimize future campaigns. This is particularly important for AI-Powered Ad Campaigns, where machine learning algorithms can adjust your strategy in real-time based on performance data.
Media Mix Modeling
Media Mix Modeling (MMM) is a technique used to measure the impact of different marketing activities on sales. By analyzing historical data, MMM can help you understand the effectiveness of various media channels and optimize your marketing mix. For example, you might find that TV ads have a higher ROI than digital ads, allowing you to adjust your budget accordingly. This technique is particularly useful for Marketing Budget Planning, ensuring you get the most out of your marketing spend.
AI-Powered Ad Campaigns
AI-powered ad campaigns leverage machine learning algorithms to optimize your marketing efforts. These campaigns can automatically adjust bids, target audiences, and even create ad content based on performance data. Tools like Google Ads and Facebook Ads offer AI-driven features that can help you maximize your ROI. By continuously learning from data, these campaigns can adapt to changing market conditions and consumer behaviors, making them a powerful tool in your marketing arsenal.
Marketing Budget Planning
Effective marketing budget planning involves allocating resources to the most impactful activities. Use historical data and predictive analytics to forecast future performance and make informed decisions. Tools like Excel and specialized software like Allocadia can help you create detailed budget plans. By regularly reviewing and adjusting your budget based on performance data, you can ensure that your marketing efforts are both efficient and effective.
Marketing Measurement Techniques
Various techniques can be used to measure the success of your marketing efforts. These include A/B testing, surveys, and focus groups. Each technique has its strengths and weaknesses, so it's important to choose the right one for your needs. For example, A/B testing is great for comparing two versions of a webpage, while surveys can provide deeper insights into customer satisfaction. By combining multiple techniques, you can get a comprehensive view of your marketing performance and make data-driven decisions.
Conclusion
In conclusion, the analysis of the latest test group results has provided invaluable insights that can drive future strategies and optimizations. By meticulously examining both quantitative data and qualitative feedback, we have identified key areas of success and potential improvement. The iterative process of testing, analyzing, and implementing changes ensures that we continually refine our approach, leading to more effective outcomes. Leveraging past test results to formulate new hypotheses and avoid previous pitfalls further enhances our ability to achieve sustained growth and user satisfaction. As we move forward, these findings will serve as a cornerstone for informed decision-making and strategic planning.
Frequently Asked Questions
What are test group results?
Test group results refer to the data and feedback collected from a specific group of participants who are exposed to different versions of a product or service to evaluate performance, usability, or other metrics.
Why are test group results important?
Test group results are crucial because they provide insights into how different versions of a product or service perform. This helps in making informed decisions about future developments and improvements.
How do you analyze quantitative data from test group results?
Quantitative data from test group results can be analyzed by interpreting numerical data, identifying winning versions, and assessing statistical significance to determine the effectiveness of different versions.
What is the role of qualitative feedback in test group analysis?
Qualitative feedback, such as user comments, helps in understanding the reasons behind user preferences and behaviors. It provides context to the quantitative data and highlights areas for improvement.
What are common pitfalls in analyzing test group results?
Common pitfalls include avoiding confirmation bias, misinterpreting data, and overlooking qualitative feedback. These can lead to incorrect conclusions and ineffective decisions.
How can test group results be applied to future experiments?
Test group results can be used to formulate new hypotheses, implement changes based on findings, and learn from failed tests. This iterative process helps in continuously improving the product or service.